In today’s globalized world, the demand for translation and localization services has never been higher. As businesses expand internationally and digital communication continues to break down language barriers, the translation industry plays a pivotal role in facilitating cross-cultural communication.
However, the landscape of the localization industry is undergoing a profound transformation thanks to advancements in technology, particularly the emergence of Chat GPT (Generative Pre-trained Transformer) and Language Learning models. Chat GPT and Language Learning models represent a paradigm shift in translation and language services. These models, powered by artificial intelligence (AI) and deep learning techniques, have the potential to revolutionize the way we approach translation tasks.
These innovations are reshaping the future of the translation industry and redefining the roles of translators, interpreters, businesses, and other stakeholders.
These innovations are reshaping the future of the translation industry and what it means for translators, interpreters, businesses, and localization industry stakeholders.
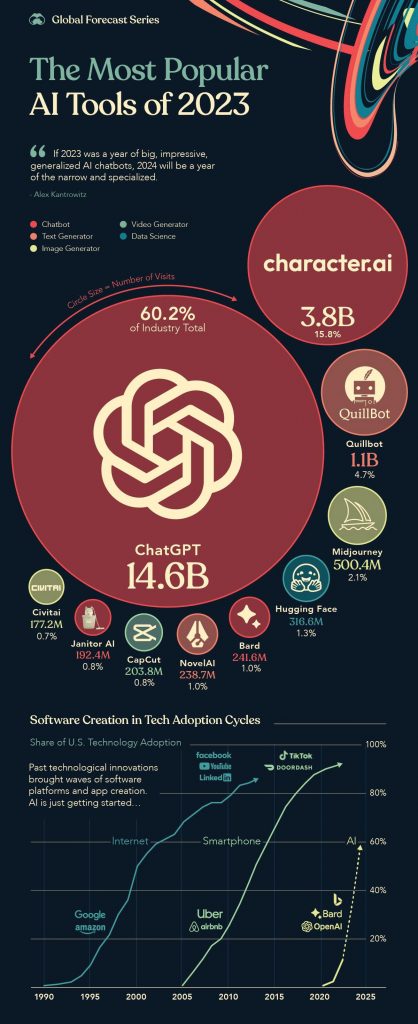
Infographics courtesy of 2024 Global Forecast Series Report, Visualcapitalist.com
Different Approaches
Chat GPT and Google Translation are both language-related AI tools, but they have different approaches, strengths, and limitations when it comes to translation tasks. Google Translate is a dedicated machine translation service that employs a statistical approach, neural machine translation (NMT), and large datasets to translate text from one language to another. It relies on pre-trained models and is designed specifically for accurate and fluent translations, supporting a wide range of languages.
In contrast, Chat GPT is a more general-purpose language model designed for text generation and understanding. While it can perform translation tasks, it doesn’t have the same level of specialization as Google Translate. Chat GPT relies on its general language understanding capabilities and context-based generation to provide translations.
Google supports a wider range of languages than AI and is tailored specifically for translation, making it more robust than AI because of its specialization. However, it still relies heavily on a database of words, representing a ‘conduit model.’ Neural machine translation (NMT) has evolved from earlier machine translation methods like Rule-based machine translation (RBMT) and Statistical Machine Translation (SMT), but it arguably remains a ‘conduit model’ that translates word by word without considering context, nuances, metaphors, or idioms. Therefore, Google’s translation may not capture the nuances or context of a sentence, making it insufficient in many professional contexts.
For instance, the auto giant Ford discovered in Belgium that when they attempted to entice customers with a marketing slogan that said ‘Every car has a high-quality body,’ it was mistranslated by Google Translate to ‘Every car has a high-quality corpse,’ illustrating the importance of context in translation.
Chat GPT vs Google Translate vs Human Translation – A fair comparison
Google Translate’s primary goal is to maintain translation fidelity, so it may not generate translations that are creatively rephrased, as Chat GPT does. Chat GPT’s translations tend to be less formal and more creatively rephrased than Google Translate. While Chat GPT can better understand context when described through AI prompts and can generate translations that take context into account, its output is less formal and flexible in nature, supports only some languages, and is used for a broader range of language-related tasks beyond pure translation. Chat GPT renders different result each time and makes mistakes and then apologizes for its own mistakes if being asked (!). This makes the output less predictable than traditional Google Translation.
Both Google Translate and Chat GPT can perform translation tasks, but their strengths and purposes differ. Google Translate specializes in accurate word-by-word translation, whereas Chat GPT offers more versatility and context-awareness, making it suitable for a broader range of language-related tasks beyond pure translation. The choice between them depends on the specific needs and priorities of the user.
Translation Quality – The verdict Chat GPT is an impressive platform that challenges Google’s word-by-word conduit model. It offers a more creative output but at the expense of being less formal, making it suitable for tasks like understanding subjects or combining documents rather than serving as a stable machine translation solution, for which Google Translate was built.
Human Translation – The GOLD Standard and Best of Both Worlds
The future of the translation industry is undoubtedly intertwined with the rise of Chat GPT and Language Learning models. While these technologies bring about both opportunities and challenges, they are ultimately tools that can enhance the work of translators, interpreters, and businesses alike. As the industry evolves, it is essential for all stakeholders to embrace innovation and collaborate to harness the full potential of these transformative technologies.
Translators and editors who utilize localization methodologies, such as file management platforms throughout the project management life cycle and translation process, known as CAT Tools (distinct from Machine Translation or AI), Translation Memories, Do-Not-Translate (DNT) Lists, Glossaries and Termbases, style guides, and translators who possess expertise in the industry subject matter, as well as being native speakers who review prior translations for clients, can focus on the finer nuances of language at a higher level and provide a superior level of translation output.
The translation industry stands at the threshold of an exciting era where language barriers are no longer insurmountable obstacles. With the right approach, collaboration, and adaptation, we can ensure that the future of translation and localization remains bright and promising.
Bibliography:
- 2024 Global Forecast Series Report, Visual Capitalist
- Interpreters vs Machines. 2020 (Jonathan Downie)
- International Journal of Communication, Jonathan Downie, Interpreters Vs Machines
- Statistical vs. Rule-Based Machine Translation S Sreelekha · 2018